As we stand on the cusp of 2024, peering into the future of artificial intelligence and large language models (LLMs), the trajectory appears both dynamic and transformative. The ever-evolving landscape of technology, with the imminent prospect of GPT-5 introducing video capabilities, is poised to redefine industries across the spectrum. From healthcare to entertainment, finance to education, the influence of LLMs continues to permeate. It promises novel horizons for diverse business domains.
LLMs in 2023
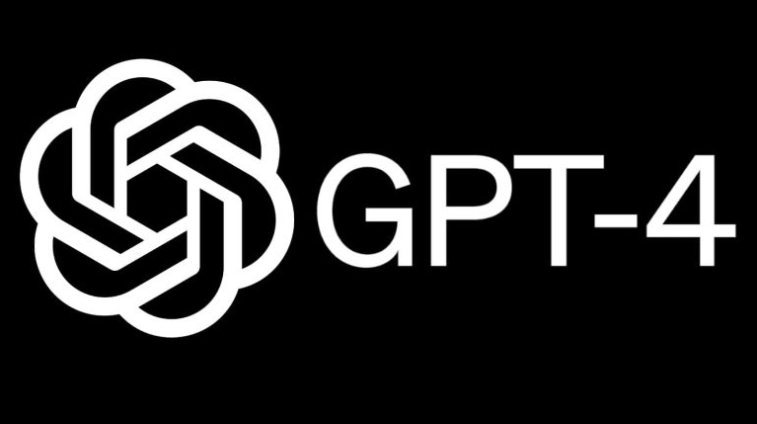
As of 2023, large language models (LLMs) stand at the zenith of scale and sophistication. Take OpenAI’s GPT-4, a behemoth with 1.7 trillion parameters, placing it among the largest and most intricate models in existence. These models undergo initial training on vast troves of internet text. The developers are also fine-tuning for specialized tasks such as translation or text completion. This fine-tuning process prioritizes performance and tailors the model to distinct applications.
Human feedback has emerged as a linchpin in the evolution of LLMs. It has elevated their accuracy, coherence, and alignment with human values. From answering questions to crafting articles, translating languages, and even generating creative content, LLMs showcase remarkable versatility.
Yet, challenges loom large. The colossal size of these models necessitates substantial computational power for both training and operation. Rigorous evaluation datasets play a pivotal role in ensuring fairness and reliability in text generation and analysis. Meanwhile, ethical considerations surrounding biases in training data and the interpretability of these models remain pressing concerns.
In this article, we will explore AI’s impact and anticipate the significant developments that lie ahead in the realm of LLMs.
Emphasis on Data Excellence and Legal Considerations
Recognizing the pivotal role of training data quality in the advancement of LLMs, the future will spotlight a heightened focus on data excellence, transparency, and legal dimensions like licensing and privacy. Ensuring high-quality, trustworthy data will be paramount for the optimal performance of generative AI, treating data as a crucial product in itself.
Shift Toward Localized Consumer Hardware Deployment
A transformative shift is underway with the rise of smaller, more efficient language models (SLMs). Positioned as potential game-changers, these models are crafted for deployment on devices with constrained processing capabilities, such as mobile devices, and hold promise for compatibility with consumer hardware like the latest processors from Apple. SLMs bring versatility and efficiency, enabling deployment on edge devices while delivering domain-specific optimizations for distinct tasks.
Diverse Deployments
In 2024, large language models (LLMs) are set to see deployment in three distinct manners:
- Expansive cloud models
- Finely tuned enterprise models
- Streamlined mobile models
The cloud will continue to host the most potent general models. They will benefit from increased inference hardware memory to accommodate even larger foundational models. Some will even approach a substantial 10 trillion parameters through innovative, sparse approaches.
A notable trend for the coming decade is the rise of enterprise LLMs. Leveraging proprietary datasets, sectors like finance can enhance fraud detection and tailor customer service by training LLMs specifically on industry-specific information. Similarly, in healthcare, LLMs trained on anonymized patient records could significantly improve disease diagnosis and treatment plan generation.
A groundbreaking development is the native integration of LLMs into phones, starting in 2024. Advances in efficient model training, exemplified by models like BTLM and Phi, enable these smaller models to match or even surpass state-of-the-art models from the previous year. Qualcomm’s demonstration of 10 billion parameter models running on Snapdragon processors paves the way for high-performance models like Mistral-7B to operate on phones independently, reducing reliance on cloud services. This marks a substantial stride in democratizing AI access, minimizing inference costs, and enhancing user privacy by processing data directly on devices.
Rise of Specialized Small Models
Microsoft’s PHI-2, a 2.7 billion-parameter model, exemplifies the trend of smaller models matching the performance of larger counterparts through strategic training data selection and innovative scaling methods. This challenges the notion that larger models always equate to superior performance.
In exploring the capabilities of smaller-scale language models (SLMs), the focus is on achieving competitive performance by leveraging high-quality training data and unique scaling techniques. Two key insights drive the success of Phi-2:
Data Quality Matters
By emphasizing the significance of training data, Phi-2’s performance is optimized by leveraging “textbook-quality” data. Synthetic datasets are crafted to impart common-sense reasoning and general knowledge, supplemented by carefully selected and filtered web data with educational value.
Innovative Scaling
Starting with the 1.3 billion parameter model Phi-1.5, knowledge transfer techniques scale up Phi-2 to 2.7 billion parameters. This approach not only expedites training but also enhances benchmark scores.
Performance Highlights of Phi-2:
- With only 2.7 billion parameters, Phi-2 outperforms Mistral and Llama-2 models with 7B and 13B parameters across various benchmarks
- Surpassing a 25x larger Llama-2-70B model in multi-step reasoning tasks, such as coding and math
- Matching or outperforming the recently-announced Google Gemini Nano 2, despite its smaller size
Open-Source LLMs
The realm of open-source large language models (LLMs) is gaining momentum. They offer a range of advantages, such as heightened data security, cost-effectiveness, privacy, and community collaboration.
LLaMA 2
In a departure from the norm, Meta has embraced openness by releasing its powerful Large Language Model Meta AI (LLaMA) and its upgraded version, LLaMA 2. Meta launched LLaMA 2 in July 2023. It is a pre-trained generative text model with 7 to 70 billion parameters. The developers fine-tuned it with Reinforcement Learning from Human Feedback (RLHF). Its versatility extends to chatbot applications and various natural language generation tasks, including programming. Meta has introduced two open versions, Llama Chat and Code Llama.
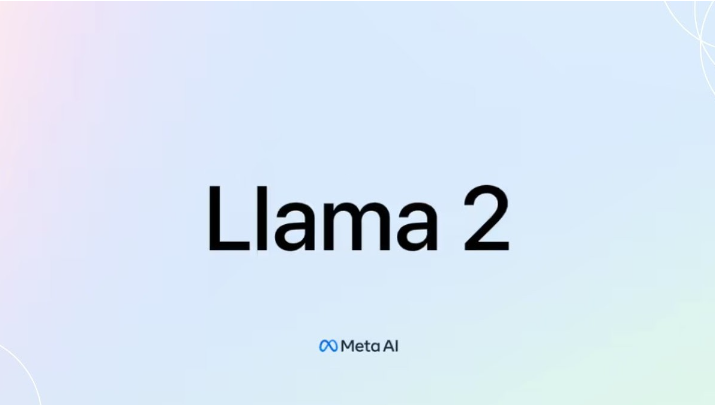
Falcon 180B
Released by the Technology Innovation Institute of the United Arab Emirates in September 2023, Falcon 180B, trained on 180 billion parameters and 3.5 trillion tokens, has surpassed benchmarks in various Natural Language Processing (NLP) tasks. Despite its free availability for commercial and research purposes, it demands substantial computing resources.
OPT-175B
Meta’s Open Pre-trained Transformers Language Models (OPT), introduced in 2022, includes OPT-175B, a powerful open-source LLM. Comparable in performance to GPT-3, it is released under a non-commercial license, limiting its use to research applications.
XGen-7B
Salesforce entered the LLM arena with XGen-7B in July 2023. Prioritizing longer context windows, XGen-7B offers an 8K context window, utilizing 7 billion parameters for training. Despite its relatively compact size, XGen delivers impressive results and is available for both commercial and research purposes.
GPT-NeoX and GPT-J
Developed by EleutherAI, GPT-NeoX (20 billion parameters) and GPT-J (6 billion parameters) present open-source alternatives to GPT. Trained with diverse datasets, they cover various domains and are available for free through the NLP Cloud API.
So, LLMs are prepared to go to the next level in 2024. Companies should get on board so that their work does not feel updated or obsolete.
Curious about enhancing your business efficiency with LLMs? Let’s explore possibilities together. Reach out today for a free consultation and elevate your operations.